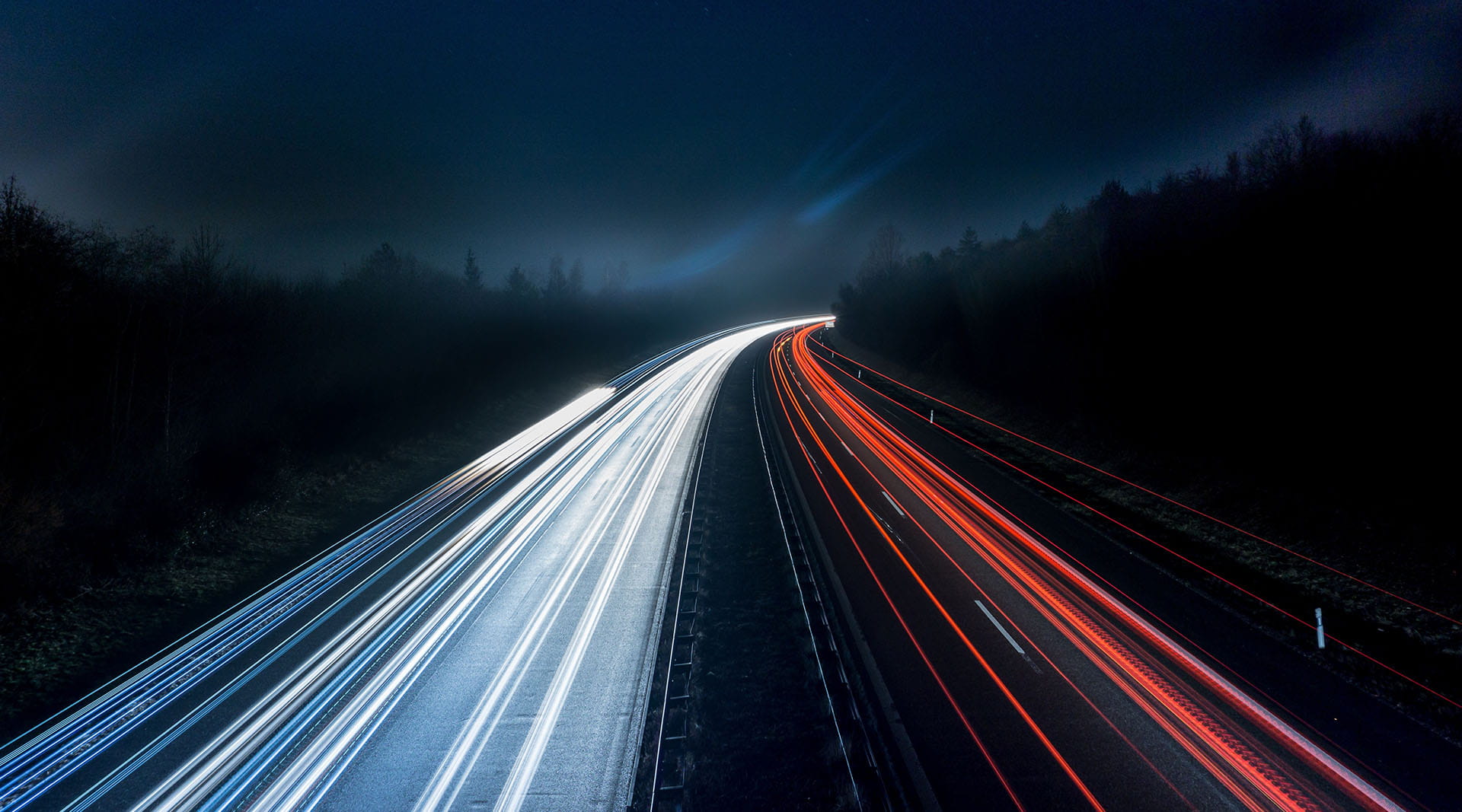
Ashurst Advance
Ashurst Advance is our NewLaw division and our expert global team delivers innovative and technology-enabled legal services to our clients across the world.
At Ashurst, we know our clients expect their legal advisers to provide innovative and cost-effective solutions to their legal and business challenges.
Leveraging our deep experience across delivery, managed services, digital, legal operations and flexible resourcing, we work seamlessly with our legal advisory and risk consulting teams to provide fully integrated solutions that are tailored to your needs and that aim to address your most complex legal challenges.
We invest in digital tools and use the latest process design methodologies to strive to:
- ensure the highest levels of quality and efficiency
- drive real value for your business.
Our experience across legal, consulting and delivery fully operationalises our legal advice and delivers innovation and real value for your business, clients and customers.
Benefits to you
We seek to understand your specific needs and tailor our solutions to meet them.
We help to save you time and money and strive to provide compelling value.
We help you to resolve your immediate challenges and to outpace change with a view to future-proofing your business.
You can have trust and confidence in Ashurst as an expert global law firm.
Explore our capabilities
Advanced Delivery
We use a powerful combination of cost-effective and flexible resourcing, technology, process design and project management to drive efficiencies and improve delivery for complex, high-volume or resource intensive work.
Legal Managed Services
An extension of your in-house legal team, we deliver efficient, quality-assured, end-to-end longer-term solutions, helping you to optimise your own resources and prioritise more strategic work.
Digital Solutions
We use the latest technology and user design principles to help streamline legal processes, optimise the delivery of legal services, drive engagement and build products that meet the challenges of a rapidly changing legal and regulatory landscape.
Legal Operations
Our Legal Operations team helps drive greater efficiency, transparency and cost certainty in large or complex matters and projects. We can also help you with your own legal operations and transformation agenda.
Flexible Resourcing
Ashurst Reach gives you access to our extensive network of highly experienced contract lawyers and paralegals with the expertise you need for all your short- to medium-term requirements.
Ashurst Advance's recent recognitions
Winner
Most Innovative Law Firm – APAC & Europe
Financial Times Innovative Lawyer Awards 2022
Band 1 Alternative Legal Service Providers – Global Law Firm LPO
Chambers & Partners 2023
Winner
Innovation in Multidisciplinary Teams
Financial Times Innovative Lawyer Awards 2022
Winner
Best Client Service Innovation
The Lawyer Awards 2021
Ashurst Advance recently celebrated its 10-year anniversary
Originally launched in Glasgow, with a small team of seven, Ashurst Advance in now a global division, with nearly 200 people working across Glasgow, London, Brisbane, Sydney, Melbourne, Perth, Abu Dhabi, Hong Kong and now Krakow.
Ashurst Advance is the global NewLaw innovation engine of the firm and a key part of what makes Ashurst so uniquely positioned through its combined offering of Legal, Risk Consulting and NewLaw services.
Play the video to find out more.
Case studies
Breach reporting managed service
A new breach reporting regime expanded the scope and introduced more stringent timeframes for the reporting of compliance events.
Large-scale Brexit and regulatory reorganisation project
Our client - a leading international bank - needed to undertake a large-scale Brexit and regulatory reorganisation.