The AI Series - How to harness the magic within financial services and remain resilient
20 September 2023
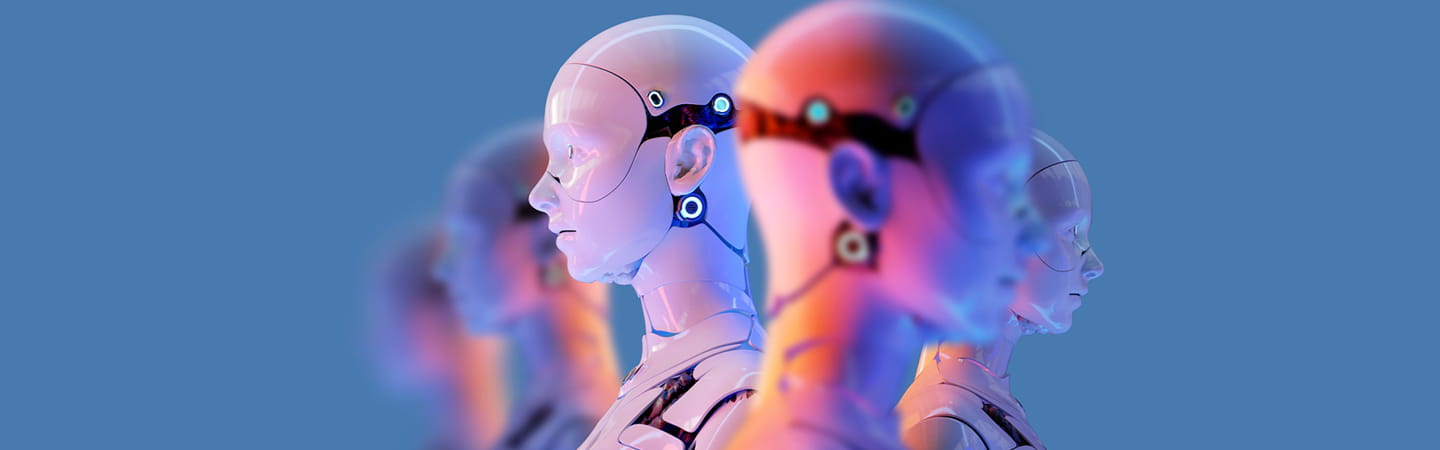
20 September 2023
Harnessing AI involves using artificial intelligence to address specific challenges, solve problems, and create new opportunities. Depending on your preferences the choices are vast and include applying machine learning, large language models, computer vision and other AI techniques to analyse data, automate tasks, make predictions, optimise processes, and improve decision making.
The clear use cases in financial services are far and wide, ranging from automating customer service with the use of chat bots to analysing vast amounts of data to create efficiencies in processes such as anti-money laundering checks, identity verification, contract analysis and credit scoring. These use cases are of course only the start. Some of the more abstract but arguably game-changing use cases include AI powered risk monitoring and assessment, data oversight, trade surveillance, fraud detection, and Internal Audit and Compliance activities.
AI is the science of making machines that can think like humans. AI technology can process vast amounts of data which allows us to:
Arguably this is the step change that has led to the increased excitement in AI.
An LLM is a deep learning algorithm that can perform a variety of natural language processing tasks. Using transformer models LLMs can analyse large datasets enabling them to recognise, translate, predict or generate text or other content.
There is no doubt that smart choices and the successful adoption of artificial intelligence will super charge the financial services industry with a whole host of new capabilities. However, the question is how to manage these new technologies in the context of the regulatory environment, where balancing innovation with legal compliance and good customer and market outcomes. This current dilemma is reinforced by the current state of flux in the regulation of AI. While the EU is legislating to implement a rules-based approach to AI governance, the UK is proposing a "contextual, sector-based regulatory framework", anchored in its existing, diffuse network of regulators and laws. As signposted in DP5/22, PRA states "the supervisory authorities may need to intervene further to manage and mitigate the potential risks and harms AI may have on consumers, firms, and the stability and integrity of the UK financial system and markets".
The savvy organisations and their development teams have realised that to remain resilient in what is likely to be a changing regulatory environment, there is an obvious need for self-policing and taking on a mindset of personal responsibility. This means responsibly cultivating data, taking an ethical approach to how data is used, and ensuring that the current legal framework on AI, which is predominantly privacy and IP based, is complied with. To support this, the framework of parameters and outcomes must be defined in a way to prevent the technology from taking the controlling role.
“The supervisory authorities may need to intervene further to manage and mitigate the potential risks and harms AI may have on consumers, firms, and the stability and integrity of the UK financial system and markets.”
Regulators and politicians are still debating governance around the ethical use of AI, however it is clear that whilst AI; provides a new methodology, there are new and emerging risks that still need to be understood, measured and managed.
Accountability for oversight should therefore sit firmly in the Boardroom and come under the Senior Managers and Certification Regime with a focus on key elements such as transparency, traceability and explainability, calibrated by stakeholder and by context. Ultimate accountability for AI does not, and should not, sit with developers and technicians.
The starting point for Senior Managers needs to focus on one simple question: do I understand the extent of the use of AI within my business? This may seem like a simple question, but the emergence of generative AI in particular has made answering this question particularly difficult. Without understanding all of the current use cases for AI, it is near impossible to adequately and holistically assess the risks it poses. In addition, where AI uses personal data, transparency obligations under data protection law oblige organisations to explain to individuals what personal data is being used and how decisions are being made. It is therefore crucial to understand who is using it, what it’s being used for, what decisions are being made and the risks this introduces to the business.
Senior Managers with accountability for the algorithmic models used by their line of business will need to demand an increased level of understanding and ensure that appropriate testing and controls have been implemented. One of the key challenges in financial services is likely to be the quality, fragmentation and incompatibility of data. Regulators will want to see evidence that effective accountability is in place, including evidence that a degree of interpretability is being provided which is appropriate to the use case and stakeholders concerned, to ensure that the outputs of an AI model are not driven by statistical quirks or resulting in discriminatory outcomes. This will also help address ethical concerns around bias in the AI algorithms and in the underlying data sets.
One of the 5 values focused cross-sectoral principles that the government in its white paper states they expect regulators to implement is “appropriate transparency and explainability”.
Arguably explainability is one of the number one challenges for this new technology. Board members of regulated entities should stringently probe what ‘sufficient’ explainability means for them and for clients, and show the confidence and integrity to admit when they do not fully understand any aspect of their firm’s use of AI. Critically, this requirement will extend to the use of third party vendors and systems, which many organisations are turning to in order to accelerate the build out of their AI capability, allowing them to keep pace with latest developments and avoid accruing legacy technologies. In these cases, accountability and responsibility will sit squarely with the regulated entity under the current regime. This presents challenges around explainability, and the lack of transparency around the sources of data used to feed those third party models. Ultimately, the use of third parties means that there is an increased risk to both operational resilience and the use of responsible AI which will need to be governed and managed very carefully.
When it comes to audits and regulators, the litmus test will be to be the ability to explain and evidence how AI models have been developed and how the decisions have been derived. Whilst the technology has obvious benefits, this alone is not going to satisfy an auditor, and therefore the right guardrails will need to be in place. If an organisation cannot explain the decision making, and does not have the right checks and balances in place to confirm that the content being generated is not biased, this will cause a challenge. Design, ongoing monitoring, and continuous improvements are therefore critical.
The requirement for human interface and triage can be an important feature to ensure oversight of the outcome. For example, in the case of large language models, which are built on probability-based weighted predictions, the machine-based answer will not necessarily be an accurate answer. The complexity around this multiplies when factoring in whether the outcome meets other important regulatory requirements such as the Consumer Duty. It is a misconception that AI will remove the need for human intervention, and in a regulated environment this is almost certainly an impossibility. Indeed, when AI is used with personal data to make decisions about individuals which have a “legal or significant effect” which is quite frequently the case in financial services, data protection law provides a right for individuals to have human intervention.
Within financial services, where dated and complicated technology stacks are all too common, "tech-debt" is something that accumulates very quickly when an organisation is looking for shortcuts to bring value to market. It is very important for organisations to set aside capacity to reduce "tech-debt" on an ongoing basis and make it an investment. This will get the necessary architectural reigns in place to quickly adapt to the new regulations as they come along.
The message for financial services firms is that we are clearly on the brink of a revolution when it comes to the magical power that is AI however, this will also impose an increased level of regulatory risk and ultimately result in a shift to the business liability profile. As the regulatory requirements around AI and the need for governance over AI increases, the organisations that survive and remain resilient without having to go back to the drawing board, will be those that architect solutions with an ethical and governance-first mindset. This philosophical approach will likely mean that when regulatory policy expands, there will be less to do, and with any luck, this approach will also bolster a global operating model.
We are not (yet) in the realms of cyborgs or Elon Musk’s brain implants but structured interaction between AI and human engagement must be the end game.
Ashurst Risk and Advisory is the consulting division of Ashurst providing risk and consulting services to complement Ashurst’s core legal services. With dedicated teams working side by side Ashurst offers truly integrated end-to-end legal and consulting capability across various risk domains including enterprise risk, governance, resilience, regulatory risk, data and AI.
In Ashurst Risk Advisory, Nisha Sanghani leads the Regulatory, Governance, Operational Risk & Resilience practice, and Matt Worsfold leads the Data & Analytics practice.
For more information visit the Risk Advisory web page.
Authors: Lee Doyle, Partner; Rhiannon Webster, Partner; Nisha Sanghani, Partner, Risk Advisory; and Matthew Worsfold, Partner, Risk Advisory.
This publication is a joint publication from Ashurst Australia and Ashurst Risk Advisory Pty Ltd, which are part of the Ashurst Group.
The Ashurst Group comprises Ashurst LLP, Ashurst Australia and their respective affiliates (including independent local partnerships, companies or other entities) which are authorised to use the name "Ashurst" or describe themselves as being affiliated with Ashurst. Some members of the Ashurst Group are limited liability entities.
The services provided by Ashurst Risk Advisory Pty Ltd do not constitute legal services or legal advice, and are not provided by Australian legal practitioners in that capacity. The laws and regulations which govern the provision of legal services in the relevant jurisdiction do not apply to the provision of non-legal services.
For more information about the Ashurst Group, which Ashurst Group entity operates in a particular country and the services offered, please visit www.ashurst.com
This material is current as at 20 September 2023 but does not take into account any developments to the law after that date. It is not intended to be a comprehensive review of all developments in the law and in practice, or to cover all aspects of those referred to, and does not constitute legal advice. The information provided is general in nature, and does not take into account and is not intended to apply to any specific issues or circumstances. Readers should take independent legal advice. No part of this publication may be reproduced by any process without prior written permission from Ashurst. While we use reasonable skill and care in the preparation of this material, we accept no liability for use of and reliance upon it by any person.
The information provided is not intended to be a comprehensive review of all developments in the law and practice, or to cover all aspects of those referred to.
Readers should take legal advice before applying it to specific issues or transactions.
Sign-up to select your areas of interest
Sign-up